Data Science in Mobile Gaming - Transforming Player Retention: A Data Science Success Story
- Website Editor
- Mar 12
- 4 min read
Updated: Mar 24
In the competitive world of mobile gaming, understanding why players leave and how to keep them engaged isn't just good business—it's essential for survival. Our recent partnership with a leading mobile game developer illustrates how sophisticated data science can transform player retention strategies and drive measurable business impact through predictive analytics.
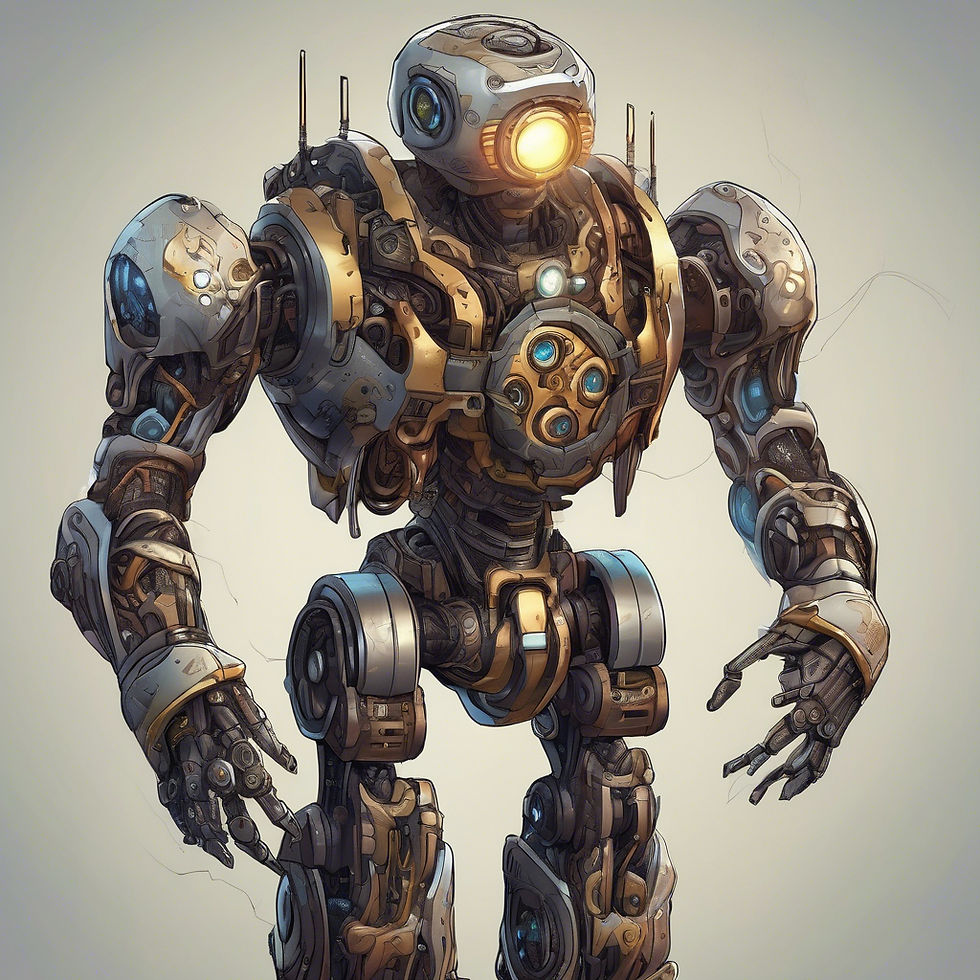
The Challenge: Predicting Player Retention in a Complex Gaming Environment
Our client, a prominent mobile game studio behind several successful puzzle games, approached us with a specific challenge: their hit puzzle game was experiencing unpredictable player drop off rates despite consistent user acquisition efforts. While the game enjoyed millions of downloads, retaining players beyond the critical 30-day mark remained elusive.
The puzzle game features unique customization options and progression mechanics that created a complex environment for understanding player behavior. Traditional retention metrics provided insight into when players were leaving but offered little clarity on why they departed or which players were at highest risk of churning.
Our Approach: From Data Exploration to Predictive Modeling
Deep Dive into User Retention and Engagement Patterns
Our data science team began with comprehensive exploration of player interaction data, analyzing:
Session frequency, duration, and time-of-day patterns
Progression milestones and difficulty curves
In-game resource management behaviors
Social feature engagement and community participation
Purchase history and virtual economy interactions
This exploration revealed several unexpected insights. For instance, contrary to conventional wisdom, difficulty spikes weren't universally driving churn—some player segments actually showed increased retention after challenging levels. However, long gaps between reward mechanisms did correlate strongly with abandonment across nearly all player types.
Advanced Feature Engineering for Predictive Power
With these initial insights, we developed a sophisticated feature engineering approach to capture the complex dynamics of player engagement:
Session-based metrics: Beyond simple counts, we created features capturing the rhythm and consistency of play patterns
Performance trajectories: Features tracking improvement or decline in player skill over time
Progression velocity: Measurements of how quickly players advance through content compared to cohort averages
Social engagement depth: Quantification of community participation and social feature utilization
Historical engagement stability: Trend features capturing the volatility in play patterns
Each feature was carefully crafted to provide predictive power while maintaining interpretability—essential for translating model insights into actionable game design changes.
Model Development with Iterative Validation
Rather than immediately building complex models at scale, we adopted an iterative approach:
Developed prototype models locally to validate feature effectiveness and establish performance baselines
Continuously refined feature sets based on both predictive power and design team feedback
Graduated to more sophisticated ensemble methods once core feature sets proved valuable
Implemented rigorous cross-validation protocols to ensure model generalizability across player cohorts
This methodical approach allowed us to maintain close collaboration with the game design team throughout development, ensuring that our models would not only predict churn accurately but would generate insights that could translate into concrete design improvements.
Technical Implementation: Building a Scalable, Production-Ready Solution
Query Optimization for Big Data Performance
The scale of data presented significant challenges—with millions of daily active users generating billions of events, computational efficiency was paramount. Our team:
Converted all feature engineering logic into highly optimized SQL queries for Snowflake execution
Created dedicated materialized views and pre-processing tables to simplify downstream analytics
Implemented smart partitioning strategies to enhance query performance
Developed incremental processing protocols to minimize redundant computations
These optimizations reduced feature generation time from hours to minutes, enabling near-real-time risk scoring for targeted interventions.
Cloud-Based Automated Pipeline Development
To ensure ongoing value, we built a fully automated machine learning pipeline using Amazon SageMaker that:
Performs daily feature updates and model scoring
Retrains models weekly to adapt to evolving player behaviors
Conducts automated performance monitoring with drift detection
Seamlessly integrates with the client's existing data infrastructure
Delivers high-risk player lists directly to intervention systems
The pipeline includes comprehensive logging, monitoring, and alerting systems to ensure reliability in production. This automation transformed churn prediction from a periodic analytical exercise into a continuous operational capability.
Results: Measurable Impact on Player Retention and Revenue
The implementation of our churn prediction system delivered significant, measurable improvements:
27% reduction in churn among identified high-risk players who received targeted interventions
18% increase in overall 30-day retention rates
32% improvement in revenue per user across previously high-risk segments
41% higher conversion rates on targeted special offers for at-risk players
Perhaps most importantly, the detailed insights from feature importance analysis led to several game design improvements that benefited all players, including:
Restructured reward timing based on optimal engagement patterns
Personalized difficulty scaling based on player skill development
Improved new player onboarding focusing on features that drive long-term retention
Beyond Gaming: Universal Principles for Customer Retention
While this project focused on mobile gaming, the approaches and techniques we employed apply broadly across industries facing customer retention challenges:
Behavior patterns over simple metrics: Looking beyond frequency to understand the quality and patterns of engagement
Personalized risk factors: Recognizing that churn drivers vary significantly across customer segments
Actionable predictions: Ensuring models don't just predict who will leave but inform what can be done to prevent it
Scalable infrastructure: Building solutions that operate continuously rather than as one-time analyses
Design feedback loops: Using predictive insights to improve the core product experience
Partnering for Retention Success
This project demonstrates how sophisticated data science can transform customer retention challenges into opportunities for product improvement and business growth. By combining deep domain expertise with advanced analytical techniques and scalable implementation, our team delivered both immediate ROI and long-term strategic value.
Whether you're facing similar challenges in gaming, e-commerce, subscription services, or any customer-centric business, the potential for predictive analytics to transform retention is substantial. The key lies not just in predicting who might leave, but in understanding why they would go and what specific actions will most effectively keep them engaged.
Our expert data science team specializes in building these kinds of high-impact, customized data science solutions—moving beyond generic analytics to deliver insights and systems that directly drive business results. Contact us today to explore how we can help transform your approach to customer retention through the power of predictive data science.
Comentarios